Artificial intelligence and financial stability
Jon Danielsson is the Director of the Systemic Risk Centre at the London School of Economics and Political Science
The financial authorities are rapidly expanding their use of artificial intelligence (AI) in financial regulation. They have no choice. Competitive pressures drive the rapid private sector expansion of AI, and the authorities must keep up if they are to remain effective.
The impact will mostly be positive. AI promises considerable benefits, such as the more efficient delivery of financial services at a lower cost. The authorities will be able to do their job better with less staff (Danielsson 2023).
Yet there are risks, particularly for financial stability (Danielsson and Uthemann 2023). The reason is that AI relies far more than humans on large amounts of data to learn from. It needs immutable objectives to follow and finds understanding strategic interactions and unknown unknowns difficult.
The criteria for evaluating the use of AI in financial regulations
We propose six questions to ask when evaluating the use of AI for regulatory purposes:
1. Does the AI engine have enough data?
2. Are the rules immutable?
3. Can we give AI clear objectives?
4. Does the authority the AI works for make decisions on its own?
5. Can we attribute responsibility for misbehaviour and mistakes?
6. Are the consequences of mistakes catastrophic?
Table 1 shows how the various objectives of regulation are affected by these criteria.
Table 1. Particular regulatory tasks and AI consequences
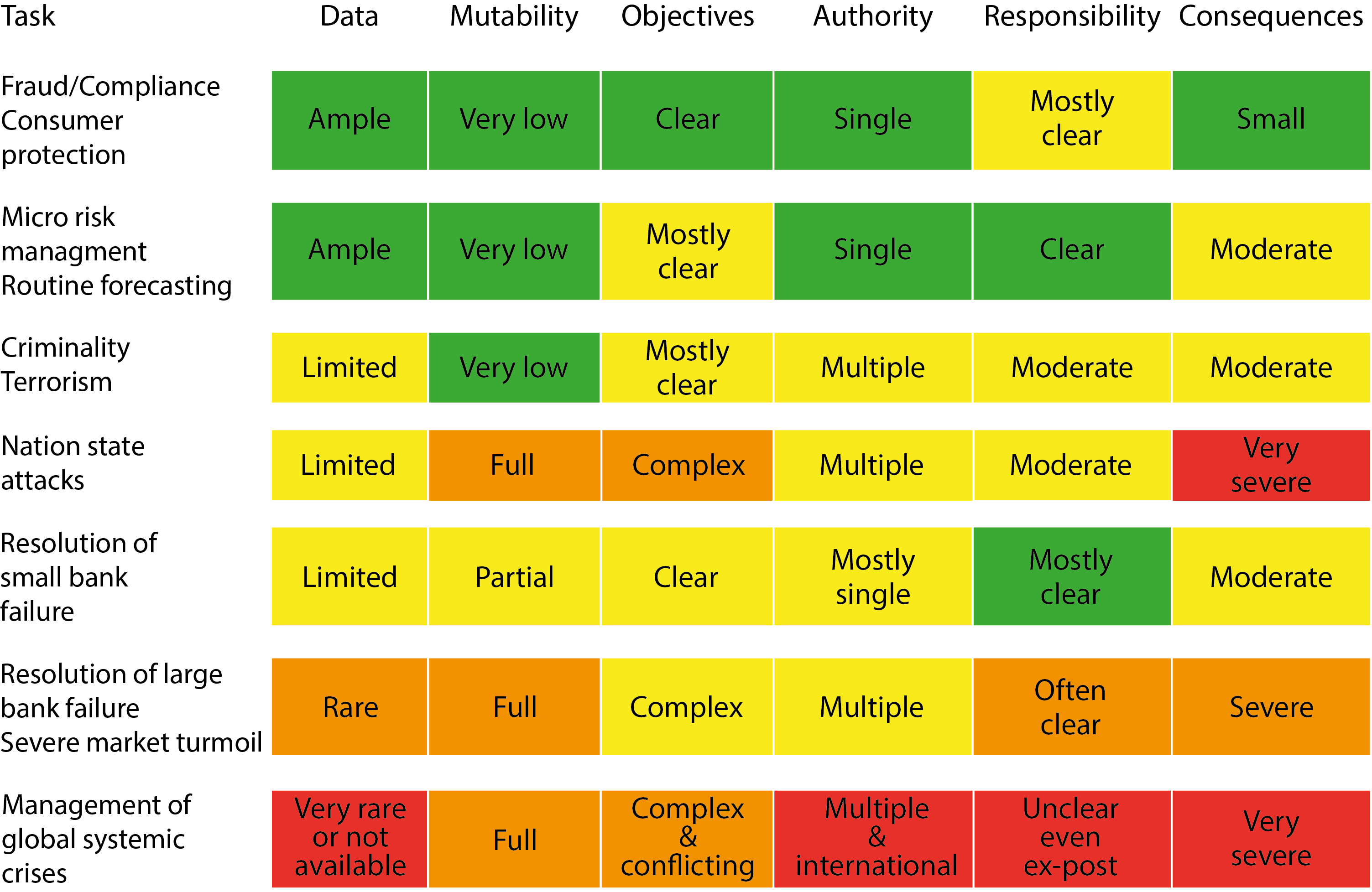
Source: Danielsson and Uthemann (2023).
Conceptual challenges
Financial crises are extremely costly. The most serious ones, classified as systemic, cost trillions of dollars. We will do everything possible to prevent them and lessen their impact if they occur, yet this is not a simple task.
Four conceptual challenges impede efforts to ensure financial stability. While frustrating human regulators, they are particularly difficult for AI to deal with.
The first conceptual challenge is data. This may seem counterintuitive because, after all, the financial system generates nearly unlimited amounts of data for AI to learn from.
However, financial data are frequently measured inconsistently, if not incorrectly. The data required for effective regulations are collected within authority silos where data sharing is limited, particularly across national boundaries.
These limitations are likely to limit and even bias the automatic learning AI requires. Some data are scarce. Laeven and Valencia’s (2018) database finds that the typical OECD country only suffers a systemic financial crisis once in 43 years.
Moreover, we usually don’t know what data are relevant until after the stress event occurs. All of this meanas that AI is at the risk of inferring an incorrect causal structure of the system.
The second challenge stems from the most serious crises that are brought about by unknown unknown events. Although all crises arise from a handful of well-understood fundamental drivers – leverage, self-preservation and complexity – the details of each are very different.
This means that crises, especially the most serious ones, are unknown unknown events and are therefore both rare and unique. Because the financial system is almost infinitely complex, the supervisors can only patrol a small portion of it.
They are likely to miss areas where the next vulnerability might emerge, although someone intent on criminal or terrorist gain might actively search for and find it.
Whereas humans have conceptual frameworks for working with infrequent, unique and unknown unknown events in a sparsely patrolled system, AI is less able to do so.
The rapid growth of AI raises significant challenges for the financial authorities. Some public sector use of AI will be very beneficial. It will improve the efficiency of most routine operations, lower costs and provide a better service to society
The third conceptual challenge relates to how the financial system reacts to control. Every time an authority makes a rule or a decision, the private sector reacts, not merely to comply but to maintain profitability while complying. That gives rise to a complex interaction between the authorities and the private sector, making the monitoring of risk and controlling financial stability challenging.
The classification in Danielsson et al (2009) of risk as either exogenous or endogenous is useful for understanding the practical consequences of the resulting feedback. Exogenous risk is based on the drivers of risk emerging from outside the financial system. Endogenous risk acknowledges that the interaction of economic agents not only causes outcomes but also modifies the structure of the financial system.
Almost every current framework for measuring financial stability risk assumes that the risk is exogenous, whereas it is virtually always endogenous. AI can be particularly misled by exogenous risk because it so strongly depends on data.
The last conceptual problem stems from how AI depends on fixed, immutable objectives. AI has to be told what to aim for. When resolving the most severe financial crises, we just don’t know the objectives beforehand, except at the most abstract level, such as preventing severe dysfunction in key financial markets and especially the failure of systemically important institution. If we knew the objectives, we would have the right laws and regulations in place, but then the crisis probably would not have happened.
Although the lack of objectives creates difficulties for human decision makers, they have a way of dealing with that – distributed decision making – which is not available to AI. Reinforcement learning might not be of much help as the problem is so complex and events rare and unique, leaving little to learn from.
Distributed decision making
The way we resolve the most serious crises is by a distributed decision-making process where all stakeholders – authorities, judiciary, private sector and political leadership – come together to make the necessary decisions. Since the objectives are only known at a high abstract level, the stakeholders do not know beforehand what the practical objectives of the process are.
Instead, they only emerge during the resolution process, and depend critically on information and interests that only emerge during it. This vital information is often implicit, putting AI at a disadvantage because it does not have an intuitive understanding of other stakeholders’ ideas and knowledge.
It is neither democratically acceptable nor prudent from a decision-making point of view to allow AI to decide on resolving crises.
Because financial crises are costly, we will go to any lengths to resolve them. The law may be changed in an emergency parliament session as Switzerland when resolving Credit Suisse. We may even suspend the law, as noted by Pistor (2013).
Rules, regulations, and the law become subordinate to the overarching purpose of crisis containment. But neither the central bank nor other financial authorities have a mandate to change or suspend the law. Only the political leadership does.
Furthermore, the resolution of a crisis will probably imply a significant redistribution of wealth. Both require democratic legitimacy, which AI does not have.
Private sector AI
Financial markets have strong complementarities that can be socially undesirable in times of stress, such as bank runs, fire sales and the hoarding of liquidity. The rapid expansion of private AI makes such outcomes more likely because, although AI is much better than humans at finding optimal solutions, it is less likely to know if they are acceptable or not.
The rapidly growing outsourcing of quantitative analysis to a small number of AI cloud vendors – Risk Management as a Service (RMaaS) as in Blackrock’s Aladdin – is further destabilising because it works to harmonise belief and action, driving procyclicality and increasing systemic risk.
Because AI will be so good at finding locally optimal solutions, it facilitates the efforts of those intent on criminal gain or terrorist damage. The authorities’ AI systems will be at a disadvantage because they have to find all vulnerabilities, whereas those intent on exploitation or damage only need to find one tiny area in which to operate. It is difficult in an infinitely complex financial system to prevent such undesirable behaviour.
The public response
The authorities need to respond to the growing price sector use of AI. A policy of not using AI for high-level decisions will probably be undermined by the stealthy adoption of AI, as there may be no feasible alternatives to it. We already use AI for many low-level tasks (Moufakkir 2023). And as we come to trust and depend on it, we will expand its use to more important domains.
Along the way, there may be little difference between AI making decisions and AI providing crucial advice. Perhaps, in a severe liquidity crisis, AI could bring together all the disparate data sources and identify all the various connections between the market participants necessary to provide the best advice for the leadership.
That is especially relevant if AI’s internal representation of the financial system is not intelligible to the human operators. What alternatives do we have to accepting the advice given if it comes from the entity doing all the monitoring and analysis?
Even if AI is explicitly prevented from making important decisions, it is likely to become highly influential by stealth because of how it affects advice given to senior decision makers, especially in times of heightened stress.
The authorities already find it difficult to prove misbehaviour when humans make decisions. That will be amplified when private sector AI makes decisions. Who will be to blame? The human manager or the AI?
When the authorities confront a private firm and it responds, ‘The AI did it, I had no idea, don’t blame me’, it creates yet another level of deniability. That facilitates the efforts of those intent on exploiting the system for private gain, legally or otherwise.
Who is accountable when AI makes decisions, and how can a regulated entity challenge them? The AI regulator may not be able to explain its reasoning or why it thinks it complies with laws and regulations. The supervisory AI will need to be overseen – regulated – differently than human supervisors.
Conclusion
The rapid growth of AI raises significant challenges for the financial authorities. Some public sector use of AI will be very beneficial. It will improve the efficiency of most routine operations, lower costs and provide a better service to society.
AI also threatens the financial system’s stability and facilitates the efforts of those intending to exploit it for criminal or terrorist purposes.
But even then, we cannot do without AI. Given the financial system’s complexity, it will probably provide essential advice to senior policymakers.
The authorities will have to respond, whether or not they want to, if they intend to remain relevant.
References
Danielsson J (2023), “When artificial Intelligence becomes a central banker”, VoxEU.org, 11 July.
Danielsson J and A Uthemann (2023), “On the use of artificial intelligence in financial regulations and the impact on financial stability”.
Danielsson J, HS Shin, and J-P Zigrand (2009), “Modelling financial turmoil through endogenous risk”, VoxEU.org, 11 March.
Danielsson J, A Uthemann and R Macrae (2020), “Artificial Intelligence as a central banker”, VoxEU.org, 6 March.
Danielsson J, R Macrae and A Uthemann (2022), “Artificial Intelligence and systemic risk”, Journal of Banking and Finance 140: 106290.
Laeven L and F Valencia (2018), “Systemic banking crises revisited”, IMF Working Paper 18/206.
Moufakkir M (2023), “Careful embrace: AI and the ECB”, technical report, European Central Bank.
Pastorello, S, G Calzolari, V Denicolo and E Calvano (2019), “Artificial Intelligence, algorithmic pricing, and collusion”, VoxEU.org, 3 February.
Pistor K (2013), “A legal theory of finance”, Comparative Journal of Economics 41(2).
This article was originally published on VoxEU.org.