New technologies in the workplace
Laura Nurski is a Research Expert at the Centre of Expertise for Labour Market Monitoring at the Faculty of Business and Economics of KU Leuven, and a Non-Resident fellow at Bruegel
As Bruegel’s Future of Work and Inclusive Growth (FWIG) project is coming to an end this year, we wish to present its main research results in a series of summaries. The focus of this summary is on Workstream 1: New technologies in the workplace. This worksteam documented how the slow but steady uptake of artificial intelligence (AI) in Europe impacts the quantity, quality and nature of jobs as well as worker wellbeing.
Uptake: the adoption and acceptance of AI in the workplace
In a policy brief (30 November 2021) and an article on the Bruegel blog (6 December 2021) Mia Hoffmann and Laura Nurski wrote about the level and drivers of AI adoption by European firms. Although estimates vary widely because of uneven data collection and lack of a standard definition and taxonomy, AI research, development and adoption in Europe is low compared to the US and China.
European firms experience barriers to adoption in terms of human capital, data availability and funding. To accelerate the roll-out of AI technology across the European Union, policymakers should alleviate those barriers, both in the environmental context – labour market, financial market and regulation – and in the technological context – data availability, basic digitisation of businesses and technological uncertainty.
Figure 1. Comparing outcomes for AI success in the EU, the US and China
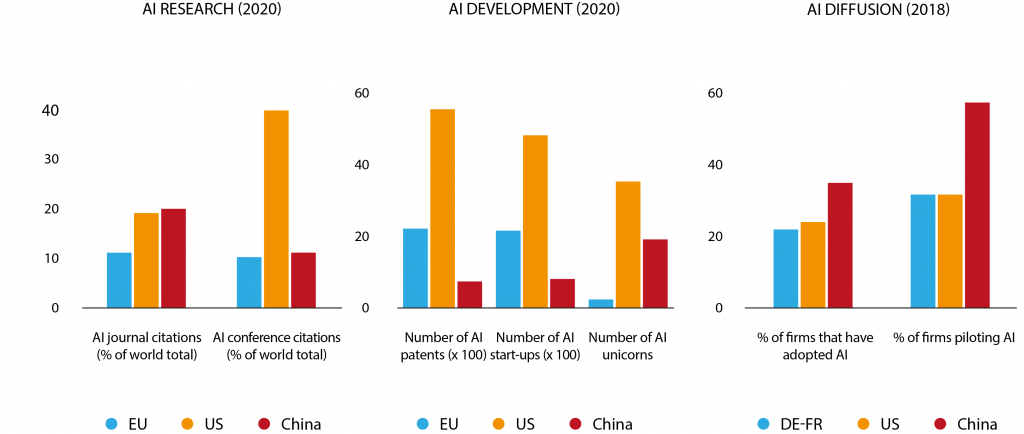
Note: a) Patents: Only ~8% of patents filed provide information on the geographical affiliation, therefore the data presented here has limited reliability. As a share of all global AI patent filings, the US accounts for 3.2%, the EU27 for 1.3% and China for 0.4%. b) Start-ups: the number reflects the sum of start-ups that received funding of at least $400,000 over the past five years (2016-2021).
Source: Bruegel based on Duranton et al (2018), CBInsights and Zhang et al (2021). Reproduced from Hoffmann & Nurski (2021).
To address the lack of a standard definition and taxonomy of AI in the workplace, Mario Mariniello and Mia Hoffmann proposed a use-based taxonomy of biometric technologies at work in their policy brief from 17 November 2021. They defined biometric technologies as AI technologies that rely on biometric data to derive inferences about the individual whose data is collected.
Their proposed taxonomy classifies technologies into four broad categories based on their main function: 1) security, 2) recruitment, 3) monitoring and 4) safety and well-being. EU regulation of AI in the workplace should integrate more detail on technology use and policymakers should design incentive mechanisms to encourage adoption of the technologies with the greatest potential to benefit workers. Bigger companies that use biometrics should be required to assess the effect of AI adoption with the active participation of their workforces.
Table 1. A taxonomy for biometric AI systems in the workplace
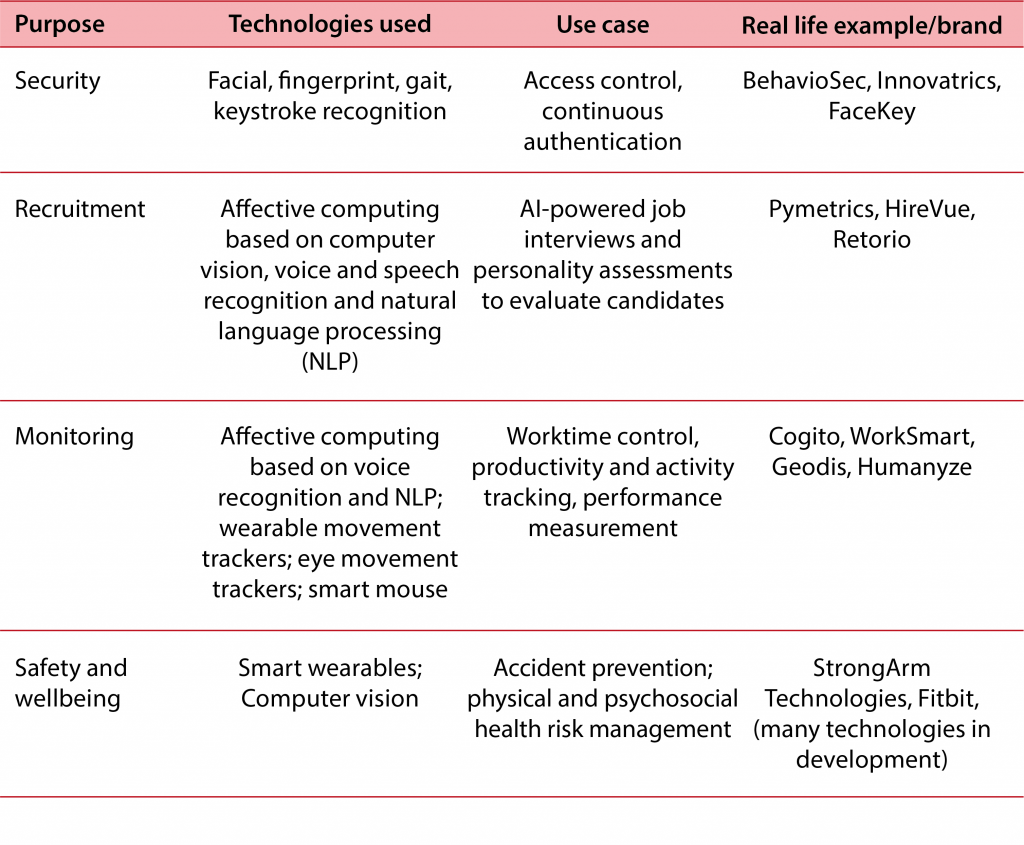
Source: Reproduced from Mariniello & Hoffmann (2021).
Active participation of workers in the implementation of workplace AI is also necessary for a smooth integration of AI in tasks and work processes, as Mia Hoffmann and Laura Nurski argued in a blog post from 30 June 2021. When new technologies are not perceived by users to be easy to use or valuable to their daily tasks, they will not be fully accepted and embraced by workers.
Employers, AI developers and regulators should ensure new technologies work for employees both before and after implementation. While post-implementation training can only go so far, essential pre-implementation interventions include increasing the system’s transparency and fairness, end-user customisation and testing, and incentive alignment between technology use and job requirements.
Laura Nurski’s case study, published on 16 March 2023, delves into the drivers and barriers concerning the adoption of artificial intelligence in the public sector.
Through examining organisational and worker acceptance of AI, the study finds the importance of a human-centred approach for successful AI adoption. This involves early involvement of workers in development phases, alignment of human resources, IT, and business processes, and targeted subsidy support mechanisms.
However, the adaptation challenges of existing work processes and legacy IT systems, alongside the varying usefulness of AI based on task routineness and worker experience, highlight the need for further investments in organizational capital for a successful AI roll-out in Europe?
Finally, in the technology adoption dashboard of 11 September 2023, Giulia Gotti, Duygu Güner and Tom Schraepen presented several key trends and figures on technology adoption in Europe. The dashboard helps users to answer questions relating to various aspects of technology adoption in the EU member states, bringing together a variety of data sources.
It provided statistics on the adoption of AI, big data applications, cloud computing and robots, as well as the necessary foundations of technology adoption, namely the availability of human resources and connectivity in Europe.
Worker participation is important for securing good job quality outcomes, at both the innovation and adoption stages
Quantity: the employment effects of AI
Georgios Petropoulos and Sybrand Brekelmans (a Bruegel blog article, 29 June 2020) evaluate AI’s differential impact on jobs and employment across the skills spectrum. While previous technological revolutions (ICT and robots) caused job polarisation (the shift of employment away from middle-skilled jobs), they found that AI is highly likely to significantly alter not only middle-skilled jobs, but also low-skill employment (see figure 1).
Moreover, while the high-skilled jobs are relatively less at risk from AI and ML-induced transformation, its impact is still non-negligible for these jobs.
Figure 2. Probability of exposure to automation of different skill groups
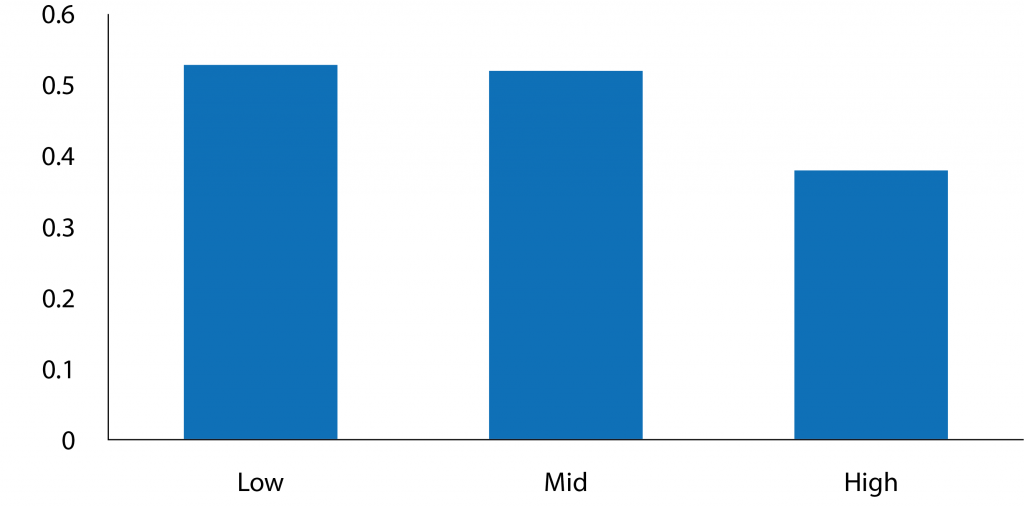
Note: This figure refers to the average exposure to automation of the individuals in each skill group. The average exposure is defined by the occupations individuals in each group have and their number. The exposure of each occupation is defined by the scale created by Nedelkoska and Quintini (2018).
Source: reproduced from Brekelmans and Petropoulos (2020).
The same authors (in a Bruegel blog article, 3 November 2020) also found that the 2007-2009 Great Recession accelerated the process of job polarisation, as the demand for middle-skilled jobs declined significantly, while the demand for both high-skilled and low-skilled jobs remained relatively stable.
This has important implications for income inequality and social mobility, as workers with middle-skilled jobs are often those with lower levels of education and training.
In both pieces, Petropoulos and Brekelmans advised policymakers to focus on supporting the re- and upskilling of workers, to help them mitigate the negative effects of job polarisation and AI.
Figure 3. Change in employment shares (%) for low-, middle- and high-skilled workers in selected EU countries for 2002, 2009 and 2016
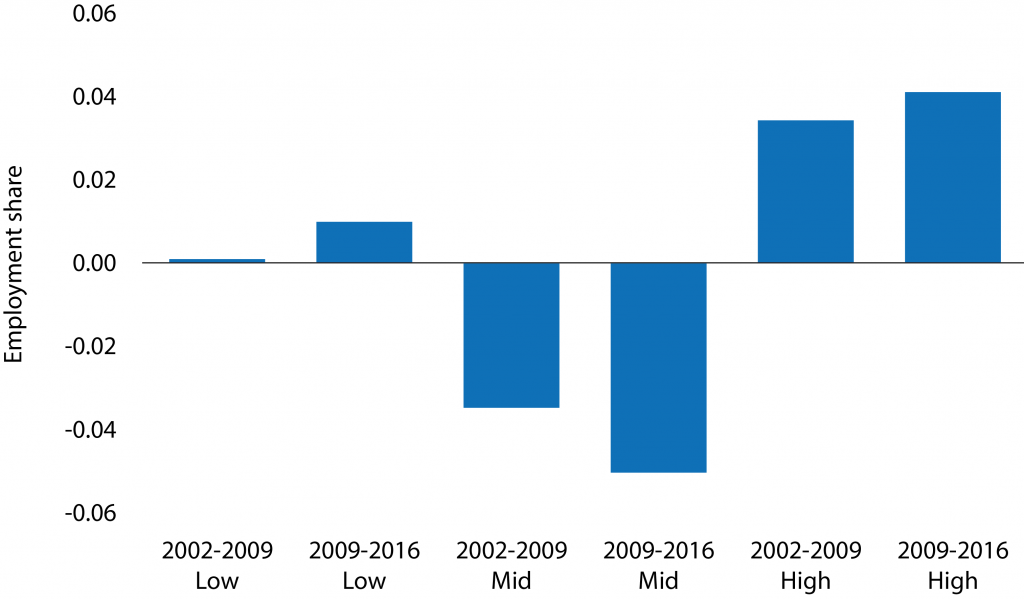
Note: For method and date refer to note of Figure 2. In this case we subtract the shares per skill level of 2002 from the shares per skill level in 2009 and shares of 2009 from the shares of 2016.
Source: Bruegel. Reproduced from Petropoulos and Brekelmans (2020).
Beyond job replacement, AI can also create new tasks and jobs. In an event on 25 May 2021 David Autor (MIT) showed that 63.5% of US employment in 2018 was in job titles that had not yet existed in 1940. Over that period, employment shifted from blue-collar and office work to technical, professional and low-paid services.
While exposure to automation did not predict the emergence of new job titles and employment growth, occupational exposure to augmentation does. David concludes that task displacement and task creation occur simultaneously with opposing consequences for employment and wage growth.
Discussants Maarten Goos (Utrecht University) and Barbara Kauffmann (DG Employment) elaborated on the policy implications of augmenting, instead of replacing, jobs: stimulating and directing technological innovation, protecting competition, and facilitating occupational and geographic mobility.
Finally, they stressed the importance of redefining labour relations to protect job quality, wages and social dialogue in the new world of work.
In a somewhat heterodox opinion Aaron Benanav and Alexis Moraitis argued in a podcast published on 19 July 2021 that the decline in labour demand is not caused by automation, but rather by the stagnation of the global capitalist economy.
Globalisation of the post-war manufacturing sector created an ‘industrial overcapacity’, pushing down prices and profit rates. While the manufacturing sector has exhausted its dynamism, the service sector – which rapidly increased in size but has less productivity growth – mostly offers precarious and underpaid jobs.
Applications of algorithmic management in the service sector put further pressure on workers. In this context, Benanav argues that Universal Basic Income (UBI) will not have strong leverage, thus other policy responses should be investigated.
In a policy brief from 5 October 2021 Rebecca Christie considered the potential for a ‘robot tax’ on companies that replace employees with automated systems. As a rallying concept for targeted levies, a robot tax should target finance and other data-driven sectors as well as traditional manufacturing and mining automation, where it can compensate for distortions due to shifts from human-driven to capital-intensive production.
Reasonable expectations should be set on the amount, time, and scale on which such a tax can raise revenue. Waivers and exemptions for small and more innovative firms should be put in place to protect innovation. Finally, any new tax on employers needs to fit with broader discussions of the corporate fair share.
At the Bruegel Annual Meetings on 6 September 2022, Laura Nurski discussed AI’s labour market impact with Brando Benifei (European Parliament), William Carter (Google), Katya Klinova (Partnership on AI). As labour market outcomes of AI innovation are shaped both during R&D and during implementation inside organisations, policy should support both an ethical development of AI as well as a responsible use in the workplace.
Katya argued that AI tends to focus excessively on automation, but policy can design mechanisms to steer innovation in a labour-complementing way. Will talked about Google’s responsible AI principles and their implementation and governance in the Google organisation.
Finally, Brando shed light on his proposed amendments to the AI act aimed to protect workers not only from discrimination, but also in terms of wages, working conditions and employment.
Quality: the job quality effects of AI
When exploring the changing nature of work in the context of technological change, one cannot ignore the impact of technology on job quality. Workstream 4 investigated the concept, dimensionality and measurement of job quality as well as its relationship to burnout. In this workstream, we assessed the specific impact of AI on the quality of work.
Laura Nurski first explored the topic of algorithmic management in a blog published on 06 May 2021. Algorithmic management is the use of AI to assign tasks and monitor workers. It included surveillance, evaluation and the automatic implementation of decisions, without human intervention. While designed to reduce costs and ensure competitiveness, optimising efficiency comes at the expense of worker wellbeing.
Laura concludes that algorithmic management is the twenty-first century’s scientific management. To mitigate its negative effects, job quality measures should be included explicitly in health and safety risk assessments for workplace artificial-intelligence systems.
In follow-up work (a working paper published 26 July 2022 and a podcast published on 27 July 2022), Laura Nurski and Mia Hoffmann dig deeper into the link between AI and job quality.
They identify four use cases of algorithmic management that impact the design and quality of jobs: algorithmic work-method instructions; scheduling of shifts and tasks; surveillance, evaluation and discipline; and task coordination.
Their review of the evidence on automation and algorithmic management shows significant impact on job quality across a wide range of jobs and employment settings. They also illustrate how AI replicates existing power dynamics in society, risking further job-quality polarisation across socioeconomic groups.
Meaningful worker participation in the adoption of workplace AI is critical to mitigate the potentially negative effects of AI adoption on workers. Therefore, policymakers should strengthen the role of social partners in the adoption of AI technology.
Table 2. Functions of management and AI use cases
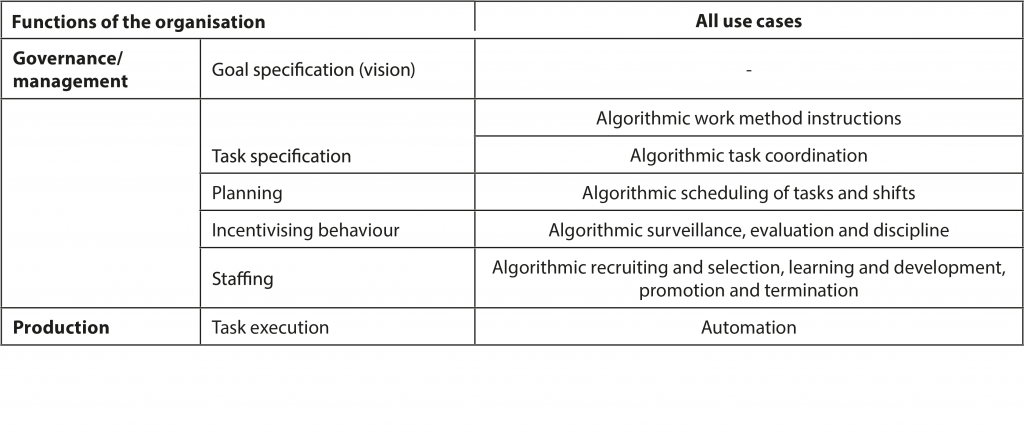
Source: Bruegel based on Cole and Kelly (2011), Martela (2019) and Puranam (2018). Reproduced from Nurski & Hoffman (2022).
The FWIG Excellence Network also contributed to the topic of digitalisation and job quality in a working paper from 22 September 2022, a podcast on 18 May 2022 and a podcast on 24 November 2021. Janine Berg, Francis Green, Laura Nurski and David Spencer examined the job quality effects of new digital technologies in Europe.
While the theoretical effects from new technologies are ambivalent across the many domains of job quality, data on robot shocks showed significant negative effects in three domains, and a positive effect in one. Some negative effects are enhanced where there is below-median collective bargaining.
The research advanced the general hypothesis that worker participation is important for securing good job quality outcomes, at both the innovation and adoption stages.
This article was originally published on Bruegel.